This seminar took place on 8 Dec 2021, 1pm.
Daniele started the seminar by explaining the motivation for his research. It is widely acknowledged that Moore’s law, the exponential scaling of classical CMOS computer technology, will cease within the next two fabrication generations due to the inability of improving the cost-effective scaling of transistors below the 3 nm channel length threshold. Simultaneously, there is increasing demand for offline real-time processing of big data, a demand that even with modern machine-learning algorithms cannot be satisfied. Daniele discussed two potential solutions: reservoir computing and stochastic computing.
Reservoir computing is a paradigm that evolves from feed forward neural networks by introducing recurrent feedback, and thus memory, to the network. Reservoir computing can be significantly more powerful than feed forward neural networks: A single reservoir can perform multiple tasks and does not require training the bulk of the network connections. It can therefore simplify pattern recognition tasks and nonlinear signal prediction.
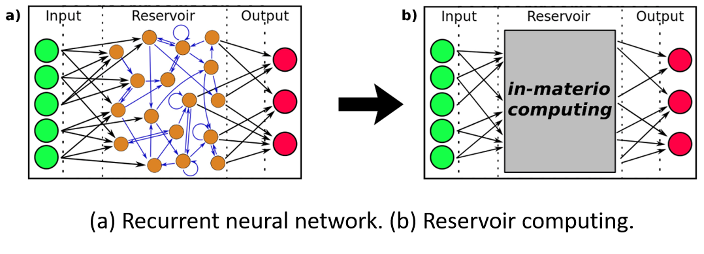
Stochastic computing is a probabilistic approach to mathematical computation that trades off deterministic accuracy for execution speed. Instead of defining operations as precise bitwise manipulations of stored finite binary numbers, one instead aims to encode numerical values as the probability of seeing a 1 or 0 in a random sequence of binary bits (known as a bitstream). With such encoding, for example, a simple and near-instantaneous AND-gate acts as a multiplication device. However, fast randomisation of input signals is needed to guarantee stochastic independence of the signals.
Daniele finally discussed a physical realisation of these two computing approaches based on skyrmions. Skyrmions are compact topological magnetic solitons that can move under electric fields and behave as nonlinear resistive elements with a delay. This makes them suitable for proof-of-principle demonstrators as nodes for reservoir computing and as randomizers for stochastic computing.
Dr Daniele Pinna is a postdoctoral researcher at the Peter Grünberg Institut, Forschungszentrum Jülich, Germany
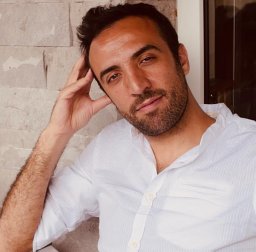
Written by Peter Horak